Drivers Nice_sys Laptops & Desktops
NIC and driver-specific statistics 2.5.4 Show the Network Status: ss # Edit source ss is a tool to dump socket statistics and replaces the netstat command.
- Drivers Nice_sys Laptops & Desktops Refurbished
- Drivers Nice_sys Laptops & Desktops Best Buy
- Drivers Nice_sys Laptops & Desktops
Feature Engineering 是把 raw data 轉換成 features 的整個過程的總稱。基本上特徵工程就是個手藝活,講求的是創造力。
本文不定期更新中。
- CPanel’s stock installation of Exim includes a Tailwatchd driver called Antirelayd. Antirelayd is a daemon that checks /var/log/maillog for POP3 and IMAP logins and keeps track of valid logins for SMTP relaying. It reads/etc/relayhosts file automatically which is dynamically-updated, so any IPs that you add to this file will not be retained.
- Download AMD Drivers & Software for Radeon, FirePro, APU, CPU, desktops, and laptops. Automatically Detect and Install Your Driver.
- Fortunately, laptops invariably provide Thermal Zone information that can be monitored and hence one can correlate CPU activity with the temperature of various components of a laptop. So last night I added Thermal Zone sampling to powerstat 0.02.00 which is enabled with the new -t option.
- CD/DVD Drivers DOS - posted in FreeDos and Dos: HiI have a job coming up on an old XP laptop with no USB booting on it.I have been busy preparing a Bootable DVD for it which autoloads my updated.
Missing Value Imputation
最簡單暴力的做法當然就是直接 drop 掉那些含有缺失值的 rows。
針對 numerical 特徵的缺失值,可以用以下方式取代:
0
,缺點是可能會混淆其他本來就是 0 的數值-999
,用某個正常情況下不會出現的數值代替,但是選得不好可能會變成異常值,要特別對待- Mean,平均數
- Median,中位數,跟平均數相比,不會被異常值干擾
針對 categorical 特徵的缺失值,可以用以下方式取代:
- Mode,眾數,最常見的值
- 改成 'Others' 之類的值
假設你要填補 age
這個特徵,然後你有其他例如 gender
這樣的特徵,你可以分別計算男性和女性的 age
的 mean、median 和 mode 來填補缺失值;更複雜一點的方式是,你可以把沒有缺失值的數據挑出來,用它們來訓練一個 regression 或 classification 模型,用這個模型來預測缺失值。
不過其實有些演算法是可以容許缺失值的,這時候可以新增一個 has_missing_value
欄位(稱為 NA indicator column)。
ref:
http://adataanalyst.com/machine-learning/comprehensive-guide-feature-engineering/
https://stats.stackexchange.com/questions/28860/why-adding-an-na-indicator-column-instead-of-value-imputation-for-randomforest
Outliers Detection
發現離群值最直觀的方式就是畫圖表,針對單一特徵可以使用 box plot;兩兩特徵則可以使用 scatter plot。
處置離群值的方式通常是直接刪除或是做變換(例如 log transformation 或 binning),當然你也可以套用處理缺失值的方式。
ref:
https://www.analyticsvidhya.com/blog/2016/01/guide-data-exploration/
https://www.douban.com/note/413022836/
Duplicate Entries Removal
duplicate 或 redundant 尤其指的是那些 features 都一樣,但是 target variable 卻不同的數據。
Feature Scaling 特徵縮放
Standardization 標準化
原始資料中,因為各個特徵的含義和單位不同,每個特徵的取值範圍可能會差異很大。例如某個二元特徵的範圍是 0 或 1,另一個價格特徵的範圍可能是 [0, 1000000],由於取值範圍相差過大導致了模型可能會更偏向於取值範圍較大的那個特徵。解決的辦法就是把各種不同 scale 的特徵轉換成同樣的 scale,稱為標準化或正規化。
狹義來說,標準化專門指的是透過計算 z-score,讓數據的 mean 為 0、 variance 為 1。
ref:
https://spark.apache.org/docs/latest/ml-features.html#standardscaler
http://scikit-learn.org/stable/modules/preprocessing.html#standardization-or-mean-removal-and-variance-scaling
https://www.quora.com/How-bad-is-it-to-standardize-dummy-variables
Normalization 歸一化、正規化
歸一化是指把每個樣本縮放到單位範數(每個樣本的範數為 1),適用於計算 dot product 或者兩個樣本之間的相似性。除了標準化、歸一化之外,其他還有透過最大、最小值,把數據的範圍縮放到 [0, 1] 或 [-1, 1] 的區間縮放法,不過這個方法容易受異常值的影響。
標準化是分別對單一特徵進行(針對 column);歸一化是對每個 observation 進行(針對 row)。
對 SVM、logistic regression 或其他使用 squared loss function 的演算法來說,需要 standardization;對 Vector Space Model 來說,需要 normalization;至於 tree-based 的演算法,基本上都不需要標準化或歸一化,它們對 scale 不敏感。
ref:
https://spark.apache.org/docs/latest/ml-features.html#normalizer
http://scikit-learn.org/stable/modules/preprocessing.html#normalization
https://www.qcloud.com/community/article/689521
Feature Transformation 特徵變換
以下適用 continuous 特徵:
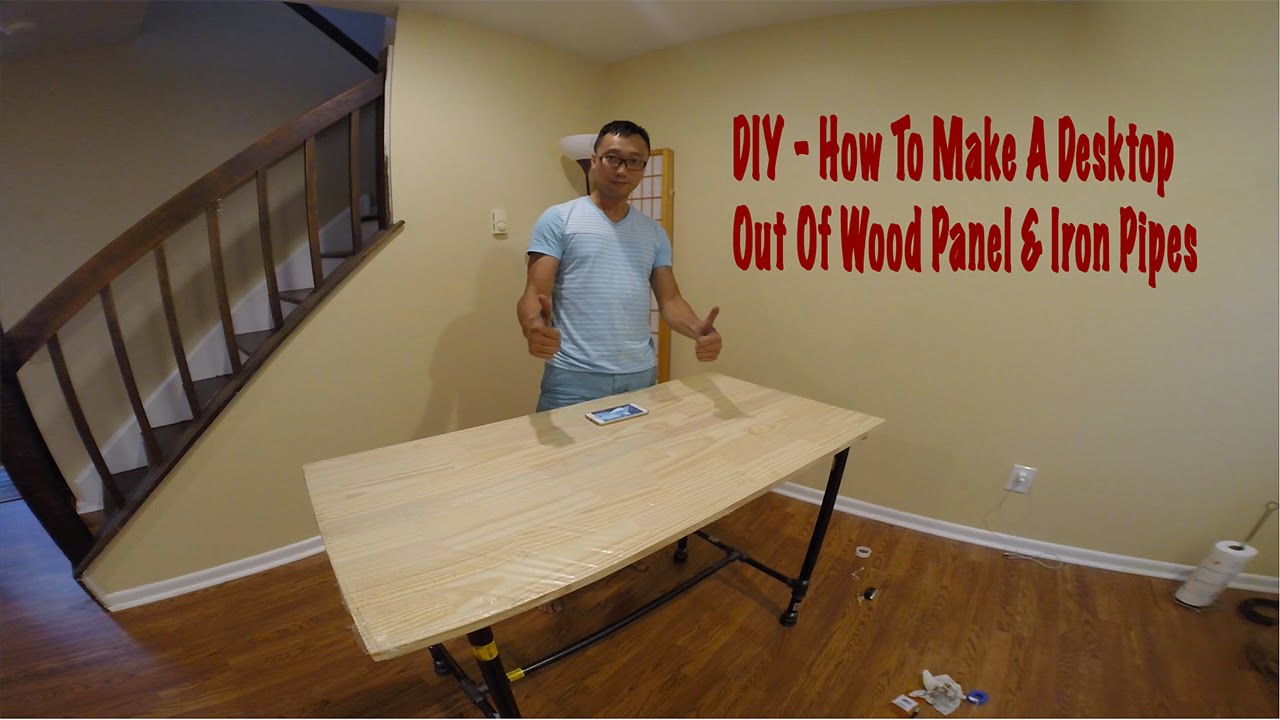
Rounding
某些精度有到小數點後第 n 位的特徵,如果你其實不需要那麼精確,可以考慮 round(value * m)
或 round(log(value))
這樣的做法,甚至可以把 round 之後的數值當成 categorical 特徵。
Log Transformation
因為 x 越大,log(x) 增長的速度就越慢,所以取 log 的意義是可以 compress 大數和 expand 小數,換句話說就是壓縮 'long tail' 和展開 'head'。假設 x 原本的範圍是 [100, 1000],log(x, 10)
之後的範圍就變成 [2, 3] 了。也常常使用 log(1 + x)
或 log(x / (1 - x))
。
另外一種類似的做法是 square root 平方根或 cube root 立方根(可以用在負數)。
ref:
https://www.safaribooksonline.com/library/view/mastering-feature-engineering/9781491953235/ch02.html
Binarization 二值化
對數值型的數據設定一個 threshold,大於就賦值為 1、小於就賦值為 0。例如 score
,如果你只關心「及格」或「不及格」,可以直接把成績對應到 1(score >= 60
)和 0(score < 60
)。或是你要做啤酒銷量分析,你可以新增一個 age >= 18
的特徵來標示出已成年。
你有一個 color
的 categorical 特徵,如果你不在乎實際上是什麼顏色的話,其實也可以改成 has_color
。
ref:
https://spark.apache.org/docs/latest/ml-features.html#binarizer
Binning
也稱為 bucketization。
以 age
這樣的特徵為例,你可以把所有年齡拆分成 n 段,0-20 歲、20-40 歲、40-60 歲等或是 0-18 歲、18-40 歲、40-70 歲等(等距或等量),然後把個別的年齡對應到某一段,假設 26 歲是對應到第二個 bucket,那新特徵的值就是 2。這種方式是人為地指定每個 bucket 的邊界值,還有另外一種拆分法是根據數據的分佈來拆,稱為 quantization 或 quantile binning,你只需要指定 bucket 的數量即可。
同樣的概念應用到其他地方,可以把 datetime 特徵拆分成上午、中午、下午和晚上;如果是 categorical 特徵,則可以先 SELECT count() ... GROUP BY
,然後把出現次數小於某個 threshold 的值改成 'Other' 之類的。或者是你有一個 occupation
特徵,如果你其實不需要非常準確的職業資訊的話,可以把 'Web Developer'、'iOS Developer' 或 'DBA' 這些個別的資料都改成 'Software Engineer'。
binarization 和 binning 都是對 continuous 特徵做 discretization 離散化,增強模型的非線性泛化能力。
ref:
https://spark.apache.org/docs/latest/ml-features.html#bucketizer
https://spark.apache.org/docs/latest/ml-features.html#quantilediscretizer
https://github.com/collectivemedia/spark-ext#optimal-binning
https://www.qcloud.com/community/article/689521
以下適用 categorical 特徵:
ref:
https://en.wikipedia.org/wiki/Categorical_variable
https://www.safaribooksonline.com/library/view/introduction-to-machine/9781449369880/ch04.html
Integer Encoding
也稱為 label encoding。
把每個 category 對應到數字,一種做法是隨機對應到 0, 1, 2, 3, 4 等數字;另外一種做法是依照該值出現的頻率大小的順序來給值,例如最常出現的值給 0,依序給 1, 2, 3 等等。如果是針對一些在某種程度上有次序的 categorical 特徵(稱為 ordinal),例如「鑽石會員」「白金會員」「黃金會員」「普通會員」,直接 mapping 成數字可能沒什麼問題,但是如果是類似 color
或 city
這樣的沒有明顯大小的特徵的話,還是用 one-hot encoding 比較合適。不過如果用的是 tree-based 的演算法就無所謂了。
有些 categorical 特徵也可能會用數字表示(例如 id),跟 continuous 特徵的差別是,數值的差異或大小對 categorical 特徵來說沒有太大的意義。
ref:
http://breezedeus.github.io/2014/11/15/breezedeus-feature-processing.html
http://phunters.lofter.com/post/86d56_194e956
One-hot Encoding (OHE)
如果某個特徵有 m 種值(例如 Taipei, Beijing, Tokyo),那它 one-hot encode 之後就會變成長度為 m 的向量:
你也可以改用 Dummy coding,這樣就只需要產生長度為 m -1 的向量:
OHE 的缺點是容易造成特徵的維度大幅增加和沒辦法處理之前沒見過的值。
ref:
http://scikit-learn.org/stable/modules/preprocessing.html#preprocessing-categorical-features
https://blog.myyellowroad.com/using-categorical-data-in-machine-learning-with-python-from-dummy-variables-to-deep-category-66041f734512
Bin-counting
例如在 Computational Advertising 中,如果你有針對每個 user 的「廣告曝光數(包含點擊和未點擊)」和「廣告點擊數」,你就可以算出每個 user 的「點擊率」,然後用這個機率來表示每個 user,反之也可以對 ad id 使用類似的做法。
ref:
https://blogs.technet.microsoft.com/machinelearning/2015/02/17/big-learning-made-easy-with-counts/
換個思路,如果你有一個 brand
的特徵,然後你可以從 user 的購買記錄中找出購買 A 品牌的人,有 70% 的人會購買 B 品牌、有 40% 的人會購買 C 品牌;購買 D 品牌的人,有 10% 的人會購買 A 品牌和 E 品牌,你可以每個品牌表示成這樣:
ref:
http://phunters.lofter.com/post/86d56_194e956
LabelCount Encoding
類似 Bin-cunting 的做法,一樣是利用現有的 count 或其他統計上的資料,差別在於 LabelCount Encoding 最後用的是次序而不是數值本身。優點是對異常值不敏感。
ref:
https://www.slideshare.net/gabrielspmoreira/feature-engineering-getting-most-out-of-data-for-predictive-models-tdc-2017/47
Count Vectorization
除了可以用在 text 特徵之外,如果你有 comma-seperated 的 categorical 特徵也可以使用這個方法。例如電影類型 genre
,裡頭的值長這樣 Action,Sci-Fi,Drama
,就可以先用 RegexTokenizer
轉成 Array('action', 'sci-fi', 'drama')
,再用 CountVectorizer
轉成 vector。
ref:
https://spark.apache.org/docs/latest/ml-features.html#countvectorizer
Feature Hashing
以 user id 為例,透過一個 hash function 把每一個 user id 映射到 (hashed1_, hashed_2, ..., hashed_m)
的某個值。指定 m << user id 的取值範圍,所以缺點是會有 collision(如果你的 model 足夠 robust,倒也是可以不管),優點是可以良好地處理之前沒見過的值和罕見的值。當然不只可以 hash 單一值,也可以 hash 一個 vector。
你可以把 feature hashing 表示為單一欄位的數值(例如 2
)或是類似 one-hot encoding 那樣的多欄位的 binary 表示法(例如 [0, 0, 1]
)。
ref:
https://github.com/apache/spark/pull/18513
https://spark.apache.org/docs/latest/ml-features.html#feature-transformation
https://www.slideshare.net/gabrielspmoreira/feature-engineering-getting-most-out-of-data-for-predictive-models-tdc-2017/42
Mean Encoding
ref:
https://zhuanlan.zhihu.com/p/26308272
Category Embedding
ref:
https://arxiv.org/abs/1604.06737
https://www.slideshare.net/HJvanVeen/feature-engineering-72376750/17
https://blog.myyellowroad.com/using-categorical-data-in-machine-learning-with-python-from-dummy-variables-to-deep-category-42fd0a43b009
User Profile 用戶畫像
使用用戶畫像來表示每個 user id,例如用戶的年齡、性別、職業、收入、居住地、偏好的各種 tag 等,把每個 user 表示成一個 feature vector。除了單一維度的特徵之外,也可以建立「用戶聽過的歌都是哪些曲風」、「用戶(30 天內)瀏覽過的文章都是什麼分類,以 TF-IDF 的方式表達。或者是把用戶所有喜歡文章對應的向量的平均值作為此用戶的 profile。比如某個用戶經常關注與推薦系統有關的文章,那麼他的 profile 中 'CB'、'CF' 和 '推薦系統' 對應的權重值就會較高。
ref:
https://mp.weixin.qq.com/s/w87-dyG9Ap9xJ_HZu0Qn-w
https://medium.com/unstructured/how-feature-engineering-can-help-you-do-well-in-a-kaggle-competition-part-i-9cc9a883514d
Rare Categorical Variables
先計算好每一種 category 的數量,然後把小於某個 threshold 的 category 都改成 'Others' 之類的值。或是使用 clustering 演算法來達到同樣的目的。你也可以直接建立一個新的 binary feature 叫做 rare,要來標示那些相對少見的資料點。
Unseen Categorical Variables
以 Spark ML 為例,當你用 training set 的資料 fit 了一個 StringIndexer
(和 OneHotEncoder
),把它拿去用在 test set 上時,有一定的機率你會遇到某些 categorical 特徵的值只在 test set 出現,所以對只見過 training set 的 transformer 來說,這些就是所謂的 unseen values。
對付 unseen values 通常有幾種做法:
- 用整個 training set + test set 來編碼 categorical 特徵
- 直接捨棄含有 unseen values 的那筆資料
- 把 unseen values 改成 'Others' 之類的已知值。
StringIndexer
的.setHandleInvalid('keep')
基本上就是這種做法
如果採用第一種方式,一但你把這個 transformer 拿到 production 去用時,無可避免地還是會遇到 unseen values。不過通常線上的 feature engineering 會有別的做法,例如事先把 user 或 item 的各項特徵都算好(定期更新或是 data 產生的時候觸發),然後以 id 為 key 存進 Redis 之類的 NoSQL 裡,model 要用的時候直接用 user id / item id 拿到處理好的 feature vector。
ref:
https://stackoverflow.com/questions/34681534/spark-ml-stringindexer-handling-unseen-labels
Large Categorical Variables
針對那種非常大的 categorical 特徵(例如 id 類的特徵),如果你用的是 logistic regression,其實可以硬上 one-hot encoding。不然就是利用上面提到的 feature hashing 或 bin counting 等方式;如果是 GBDT 的話,甚至可以直接用 id 硬上,只要 tree 足夠多。
ref:
https://www.zhihu.com/question/34819617
Feature Construction 特徵建構
特徵構建指的是從原有的特徵中,人工地創造出新的特徵,通常用來解決一般的線性模型沒辦法學到非線性特徵的問題。其中一個重點可能是能不能夠過某些辦法,在特徵中加入某些「額外的資訊」,雖然也得小心數據偏見的問題。
如果你有很多 user 購物的資料,除了可以 aggregate 得到 total spend
這樣的 feature 之外,也可以變換一下,變成 spend in last week
、spend in last month
和 spend in last year
這種可以表示「趨勢」的特徵。
範例:
author_avg_page_view
: 該文章作者的所有文章的平均瀏覽數user_visited_days_since_doc_published
: 該文章發布到該用戶訪問經過了多少天user_history_doc_sim_categories
: 用戶讀過的所有文章的分類和該篇文章的分類的 TF-IDF 的相似度user_history_doc_sim_topics
: 用戶讀過的所有文章的內文和該篇文章的內文的 TF-IDF 的相似度
ref:
https://medium.com/unstructured/how-feature-engineering-can-help-you-do-well-in-a-kaggle-competition-part-i-9cc9a883514d
https://www.safaribooksonline.com/library/view/large-scale-machine/9781785888748/ch04s02.html
https://www.slideshare.net/HJvanVeen/feature-engineering-72376750/23
Temporal Features 時間特徵
對於 date / time 類型的資料,除了轉換成 timestamp 和取出 day、month 和 year 做成新的欄位之外,也可以對 hour 做 binning(分成上午、中午、晚上之類的)或是對 day 做 binning(分成工作日、週末);或是想辦法查出該日期當天的天氣、節日或活動等訊息,例如 is_national_holiday
或 has_sport_events
。
更進一步,用 datetime 類的資料通常也可以做成 spend_hours_last_week
或 spend_money_last_week
這種可以用來表示「趨勢」的特徵。
Text Features 文字特徵
ref:
https://www.slideshare.net/HJvanVeen/feature-engineering-72376750/57
Spatial Features 地理特徵
如果你有 city
或 address
等特徵,可以新建出 latitude
和 longitude
兩個 features(當然你得透過外部的 API 或資料來源才做得到),再組合出 median_income_within_2_miles
這樣的特徵。
ref:
https://www.slideshare.net/HJvanVeen/feature-engineering-72376750/47
Cyclical Features
ref:
http://blog.davidkaleko.com/feature-engineering-cyclical-features.html
Features Interaction 特徵交互
假設你有 A
和 B
兩個 continuous 特徵,你可以用 A + B
、A - B
、A * B
或 A / B
之類的方式建立新的特徵。例如 house_age_at_purchase = house_built_date - house_purchase_date
或是 click_through_rate = n_clicks / n_impressions
。
還有一種類似的作法叫 Polynomial Expansion 多項式展開,當 degree 為 2 時,可以把 (x, y)
兩個特徵變成 (x, x * x, y, x * y, y * y)
五個特徵。
ref:
https://spark.apache.org/docs/latest/ml-features.html#polynomialexpansion
https://elitedatascience.com/feature-engineering-best-practices
Feature Combination 特徵組合
也稱為特徵交叉。
特徵組合主要是針對 categorical 特徵,特徵交互則是適用於 continuous 特徵。但是兩者的概念是差不多的,就是把兩個以上的特徵透過某種方式結合在一起,變成新的特徵。通常用來解決一般的線性模型沒辦法學到非線性特徵的問題。
假設有 gender
和 wealth
兩個特徵,分別有 2 和 3 種取值,最簡單的方式就是直接 string concatenation 組合出一個新的特徵 gender_wealth
,共有 2 x 3 = 6 種取值。因為是 categorical 特徵,可以直接對 gender_wealth
使用 StringIndexer
和 OneHotEncoder
。你當然也可以一起組合 continuous 和 categorical 特徵,例如 age_wealth
這樣的特徵,只是 vector 裡的值就不是 0 1 而是 age
本身了。
假設 C 是 categorical 特徵,N 是 continuous 特徵,以下有幾種有意義的組合:
median(N) GROUP BY C
中位數mean(N) GROUP BY C
算術平均數mode(N) GROUP BY C
眾數min(N) GROUP BY C
最小值max(N) GROUP BY C
最大值std(N) GROUP BY C
標準差var(N) GROUP BY C
方差N - median(N) GROUP BY C
ref:
http://breezedeus.github.io/2014/11/15/breezedeus-feature-processing.html
http://phunters.lofter.com/post/86d56_194e956
https://zhuanlan.zhihu.com/p/26444240
http://blog.csdn.net/mytestmy/article/details/40933235
Feature Extraction 特徵提取
通常就是指 dimensionality reduction。
- Principal Component Analysis (PCA)
- Latent Dirichlet Allocation (LDA)
- Latent Semantic Analysis (LSA)
Feature Selection 特徵選擇
特徵選擇是指透過某些方法自動地從所有的特徵中挑選出有用的特徵。
ref:
http://scikit-learn.org/stable/modules/feature_selection.html
Filter Method
採用某一種評估指標(發散性、相關性或 Information Gain 等),單獨地衡量個別特徵跟 target variable 之間的關係,常用的方法有 Chi Square Test(卡方檢驗)。這種特徵選擇方式沒有任何模型的參與。
以相關性來說,也不見得跟 target variable 的相關性越高就越好,
ref:
https://spark.apache.org/docs/latest/ml-features.html#chisqselector
http://files.cnblogs.com/files/XBWer/%E6%9C%BA%E5%99%A8%E5%AD%A6%E4%B9%A0%E3%81%AE%E7%89%B9%E5%BE%81.pdf
Wrapper Method
會採用某個模型來預測你的 target variable,把特徵選擇想成是一個組合優化的問題,想辦法找出一組特徵子集能夠讓模型的評估結果最好。缺點是太耗時間了,實務上不常用。
Drivers Nice_sys Laptops & Desktops Refurbished
ref:
http://www.cnblogs.com/heaad/archive/2011/01/02/1924088.html
Embedded Method
通常會採用一個會為特徵賦予 coefficients 或 importances 的演算法,例如 Logistic Regression(特別是使用 L1 penalty)或 GBDT,直接用權重或重要性對所有特徵排序,然後取前 n 個作為特徵子集。
ref:
http://scikit-learn.org/stable/modules/feature_selection.html#feature-selection-using-selectfrommodel
https://www.zhihu.com/question/28641663
Drivers Nice_sys Laptops & Desktops Best Buy
Feature Learning 特徵學習
也稱為 Representation Learning 或 Automated Feature Engineering。
- GBDT
- Neural Network: Restricted Boltzmann Machines
- Deep Learning: Autoencoder
ref:
https://zhuanlan.zhihu.com/p/26444240
Data Leakage 數據洩漏
就是指你在 features 中直接或間接地加入了跟 target variable 有關的數據。
ref:
https://zhuanlan.zhihu.com/p/26444240
Target Engineering
Drivers Nice_sys Laptops & Desktops
雖然不能算是 feature engineering 的一部分,但是其實你也可以對 target variable / label(就是你的模型要預測的那個值)做點變換。例如 log(y + 1)
或 exp(y) - 1
。
